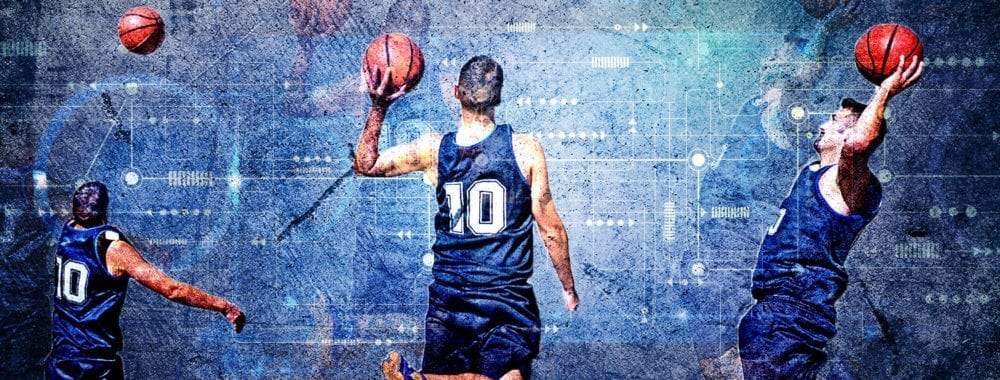
Fans regularly consume STATS’ data without knowing the original source of the information, and that isn’t necessarily changing as the company explores future applications of richer data. What is different in 2017 from 1987, 1997 or even 2007 is STATS’ specialization from often drawing the line at providing sports information to more properly contextualizing it for clients.
One of the leaders making that happen at STATS emerged from behind the scenes to speak with Sirius XM Wharton Moneyball hosts Adi Wyner and Shane Jensen earlier this month. Patrick Lucey, STATS Director of Data Science, reflected on five years of STATS SportVU tracking data collection and provided insight on the answers now coming from growing sample sizes and industry advances.
STATS began tracking NBA games with its optical system SportVU half a decade ago, which happened to coincide with what Lucey marked as a particularly interesting time in sports science.
“Something happened in 2012. Deep learning came along,” said Lucey, co-author of the 2016 MIT Sloan Sports Analytics Conference first-place research paper on patterns of player movement and ball striking in tennis.
“It’s like a perfect storm. I like to think of it as a three-legged stool. First of all, for deep learning to work, you need three things. The first thing is you need a lot of data. Luckily at STATS, we have that. The second thing is you need the computational power. … The third thing is having the people to actually know how to work or set up the network.”
For nearly two years, Lucey has sat atop that stool at STATS and helped expand player tracking into additional sports with an emphasis on the global game of football. Plenty of his current work, including his 2017 MIT Sloan Conference runner-up paper on shooting styles in basketball, has involved finding the proper ways to ask and answer the what-if questions that weren’t confrontable in STATS’ earlier years.
“The big thing – and this is what we can do really well – we can contextualize the data,” Lucey said. “…You can ask us specific questions. You can say, ‘Well, what’s the likelihood of this player making that shot? Or, what happens if I switch that player with another player?’
“So, we can really do fine-grain simulations and ask those what-if questions. … We have the box score or we have these players’ stats, but (I think the next stage in analytics) is to simulate these kinds of specific plays and see what a different player will do in these situations. In a given situation, we can model that context and we can give more precise answers because we understand the data in those situations.”
The particulars of body pose have become one of his key interests. Specifically, advances in player tracking – and the potential for more accurate predictive analysis – lies in an increased emphasis on measurements that can go beyond using a basic center of mass to establish the XY coordinates in optical tracking.
“Once upon a time, we could capture body pose, but we had to do that in a lab setting,” Lucey said. “… Now we can actually do it in the wild.”
Lucey’s team is constantly honing in on the next big thing in its field, and his view for how performance will be evaluated more closely in the future might surprise some. It’s not necessarily about creating fresh analytical metrics. It’s about the relationship between people and technology.
“I don’t actually think new metrics is the way to go,” Lucey said. “I don’t think that’s the future. I think it’s a symbiosis between a human and a computer. So can we develop new technology to help a domain expert do their job better. I think that’s really the next step in sports analytics. Just enabling, creating this kind of technology just to help coaches or analysts or people at home to be able to ask these what-if questions. I don’t think we’re that far off.”